Water saturation challenges based on cementation exponent
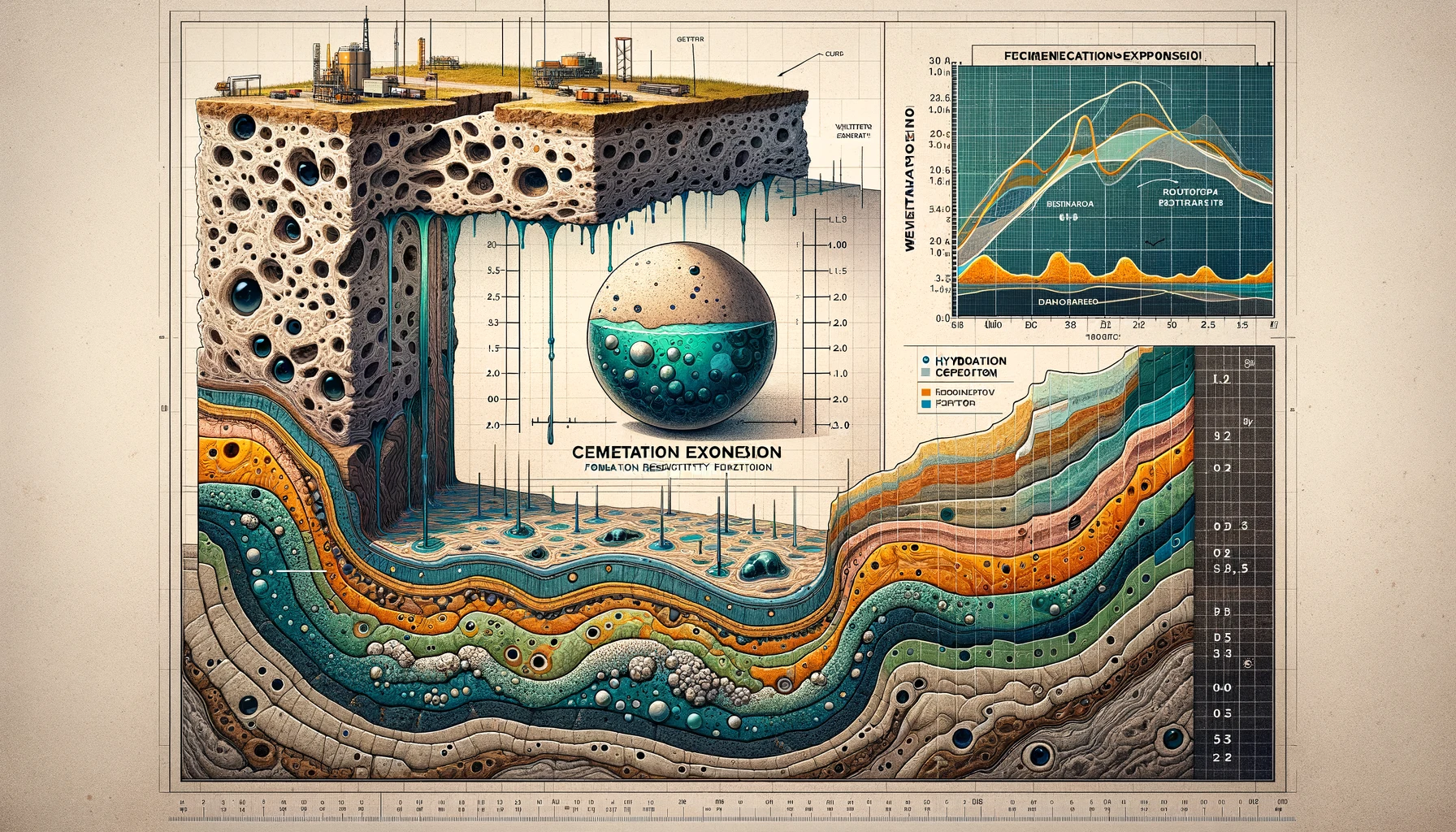
It is very challenging for any water saturation computation using the precise and actual cementation exponent m value of your reservoir. It is derived from a special core analysis based on the formation resistivity factor and porosity.
The impact of the cementation exponent on water saturation computation can change water saturation from 40% to 70% if your m value increases from 2 to 3.
It is pretty expensive to have cementation exponent in several wells in your field.
Proposed Solutions and Recommendations:
1. Predict mmm Using Machine Learning (ML):
-
Data Requirements:
- Input features: Wireline logs (e.g., porosity, resistivity, volume of shale) and interpreted logs.
- Output: Known mmm values from cored wells.
-
ML Workflow:
- Preprocess data (handle missing values, normalize features).
- Train ML models (e.g., Random Forest, Gradient Boosting, Neural Networks).
- Validate using a portion of the dataset with known mmm values.
- Apply the model to un-cored wells.
-
Advantages:
- Cost-effective and scalable.
- Models can account for relationships between logs and mmm.
2. Cross-Plots of Porosity Types:
- Use published cross-plots linking isolated pores and total porosity:
- Plot total porosity (ϕt\phi_tϕt) vs. isolated porosity (ϕi\phi_iϕi).
- Intersection lines on these plots often represent mmm values for specific formations.
- This approach works well in the absence of core data but requires expertise in interpreting cross-plots.
3. Borehole Image Logs:
-
Porosity Type Identification:
- Use borehole images to distinguish intergranular, vuggy, and fracture porosities.
- Correlate porosity types with mmm values derived from analog fields or sensitivity analysis.
-
Advanced Analysis:
- Advanced borehole image modules can quantify carbonate heterogeneity, which correlates with mmm.
-
Sensitivity Analysis:
- Perform water saturation computations across a range of mmm values.
- Match SwS_wSw results with Dean-Stark water saturation (from core data, if available) to identify the most representative mmm.
4. Dielectric Logging Tools:
- Use dielectric tools to compute continuous m/nm/nm/n curves:
- Based on bimodal carbonate interpretation models, these tools provide high-resolution estimates of mmm values.
- Integrate these curves into Archie’s equation for more accurate SwS_wSw computations.
Practical Workflow:
-
Core Wells (SCAL Data):
- Use SCAL-derived mmm values to train or validate ML models.
- Correlate with wireline and borehole image logs for understanding trends.
-
Un-Cored Wells:
- Use ML models to predict mmm based on wireline logs.
- Supplement with cross-plots or borehole image interpretations for porosity types.
-
Dielectric Logs (if available):
- Apply continuous m/nm/nm/n curves to refine water saturation estimates.
-
Validation and Sensitivity Analysis:
- Validate predicted mmm values using independent datasets or analog fields.
- Run sensitivity analysis with multiple mmm values to understand the range of water saturation and its impact on reservoir evaluation.
Key Takeaways:
- The cementation exponent mmm significantly influences water saturation calculations and reservoir characterization.
- Cost-effective alternatives to SCAL include ML-based predictions, borehole image interpretation, and dielectric tools.
- Sensitivity analysis with varying mmm values helps address uncertainties in water saturation estimates.