Oil and gas professionals work with a huge volume of information every day. This information is necessary for construction of the accurate static and dynamic reservoir models. However, significant part of the information remains unused by human “errors” and due to limited computational resources. This missing part could give significant impact to the reservoir model accuracy. Understanding of the machine learning and big data methods can help to utilize input information in the most complete way and reach the most accurate results.
This course explains and gives recommendations to more experienced participants of how to effectively apply machine learning methods in our real-life projects. it is built in order to explain the theory of machine learning methods (regression, decision trees, neural networks, support vector machines, algorithms ensembles), suggest recommendations for practical use of these methods and strategy for the most appropriate method selection.
- Analyze, preprocess and visualize big datasets in Python
- Understand machine learning basics and be able to solve problems using machine learning methods
- Use machine learning algorithms for comprehensive data analysis and be able to train accurate predictive models
- Effectively collaborate with data scientists in the project team
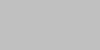
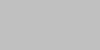
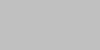
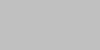
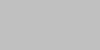
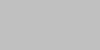
- Introduction to the course and why you should take it
- What is data analysis and machine learning specialization?
- Python language and its installation
- Introduction and working with IPyton Notebook
- Python syntax
- Python libraries (Pandas, NumPy, Scipy, Matplotlib)
- Derivative and gradient
- Vectors, matrices and operations on them
- Probability and random variable
- Distribution, density and their properties
- Basic formulas of probability theory
- Distribution types (Gaussian, Bernoulli)
- Gradient descent method
- Newton's method
- Machine learning terminology
- Machine learning problems (supervised learning, classification and regression tasks, unsupervised learning, clustering, anomaly detection, dimensionality reduction)
- Quality metrics and loss functions
- Regularization
- The problem of overfitting: bias-variance tradeoff
- Cross-validation
- Introduction to the scikit-learn library for petrophysical data analysis
- Overview of the open source data community (Kaggle, etc)
- Data preprocessing (creation and verification of characteristics, data cleaning)
- Data visualization
- Lab: data preprocessing
- Linear regression
- Lab: linear regression on petrophysical data
- Logistic regression
- Lab: logistic regression on petrophysical data
- Decision tree concept
- Lab: building a decision tree
- Random Forest
- Lab: Random Forest training on petrophysical data
- Gradient boosting
- Lab: training the decision trees ensemble on petrophysical data with XGBoost library
- Neural networks intuition
- Backpropagation
- Lab: training neural network on petrophysical data with Keras library
- Deep Learning and other types of neural networks (autoencoder, recurrent neural networks, convolutional neural networks, generative adversarial networks)
- Cluster analysis (in the context of K-Means algorithm)
- Principal Component Analysis (PCA)
- Mixture of Gaussians
- How to prepare "raw" data for learning and create new features?
- How to choose the best algorithm?
- How to configure the algorithm and choose the best set of parameters?
- How to evaluate the quality of the algorithm?
- Review of articles on machine learning for geological and sedimentological problems solution
Date | Location | Language | Price | Format | ||
---|---|---|---|---|---|---|
Currently, this course is conducted only in an intracorporate format. |
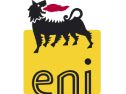
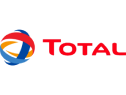
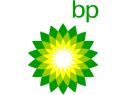
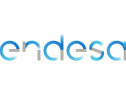
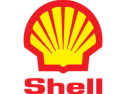
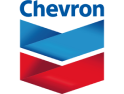
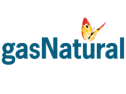
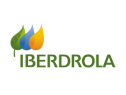
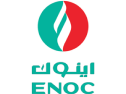
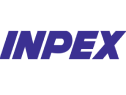
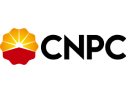
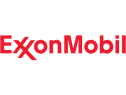
Training can take place in 4 formats:
- Self-paced
- Blended learning
- Instructor-led online (webinar)
- Instructor-led offline (classroom)
Description of training formats:
- Self-paced learning or e-Learning means you can learn in your own time and control the amount of material to consume. There is no need to complete the assignments and take the courses at the same time as other learners.
- Blended learning or "hybrid learning" means you can combine Self-paced learning or e-Learning with traditional instructor-led classroom or webinar activities. This approach requires physical presence of both teacher and student in physical or virtual (webinars) classrooms or workshops. Webinar is a seminar or presentation that takes place on the internet, allowing participants in different locations to see and hear the presenter, ask questions, and sometimes answer polls.
- Instructor-led training, or ILT, means that the learning can be delivered in a lecture or classroom format, as an interactive workshop, as a demonstration under the supervision and control of qualified trainer or instructor with the opportunity for learners to practice, or even virtually, using video-conferencing tools.
When forming groups of students, special attention is paid to important criteria - the same level of knowledge and interests among all students of the course, in order to maintain stable group dynamics during training.
Group dynamics is the development of a group in time, which is caused by the interaction of participants with each other and external influence on the group. In other words, these are the stages that the training group goes through in the process of communicating with the coach and among themselves.
The optimal group size for different types of training:
- Self-paced / E-learning: 1
- Instructor-led off-line (classroom): 6 – 12
- Instructor-led on-line (webinar): 6 – 12
- Blended learning: 6 – 12
- Workshop: 6 – 12
- On-the-job: 2 – 4
- Simulator: 1 – 2
Feedback in the form of assessments and recommendations is given to students during the course of training with the participation of an instructor and is saved in the course card and student profile.
In order to control the quality of the services provided, students can evaluate the quality and training programme. Forms of assessment of the quality of training differ for courses with the participation of an instructor and those that are held in a self-paced format.
For courses with an instructor, start and end dates are indicated. At the same time, it is important to pay attention to the deadlines for passing tests, exams and practical tasks. If the specified deadlines are missed, the student may not be allowed to complete the entire course programme.
A personal account is a space for storing your training preferences, test and exam results, grades on completed training, as well as your individual plan for professional and personal development.
Users of the personal account have access to articles and blogs in specialized areas, as well as the ability to rate the completed training and leave comments under the articles and blogs of our instructors and technical authors
Registered users of a personal account can have various roles, including the role of a student, instructor or content developer. However, for all roles, except for the student role, you will need to go through an additional verification procedure to confirm your qualifications.
Based on the results of training, students are issued a certificate of training. All training certificates fall into three main categories:
- Certificate of Attendance - students who successfully completed the course but did not pass the tests and exams can apply for a certificate of attendance.
- Certificate of Completion - students who have successfully completed a course could apply for a Certificate of Completion, this type of certificate is often required for compliance training.
- Verified Certificate - it is a verified certificate that is issued when students have passed exams under the supervision of a dedicated proctor.
You can always download a copy of your training certificate in PDF format in your personal account.
You will still have access to the course after completing it, provided that your account is active and not compromised and Tecedu is still licensed for the course. So if you want to review specific content in the course after completing it, or do it all over again, you can easily do so. In rare cases, instructors may remove their courses from the Tecedu marketplace, or we may need to remove a course from the platform for legal reasons.
During the training, you may encounter various forms of testing and knowledge testing. The most common assessment methods are:
- preliminary (base-line assessment) - to determine the current level of knowledge and adapt the personal curriculum
- intermediate - to check the progress of learning
- final - to complete training and final assessment of knowledge and skills, can be in the form of a project, testing or practical exam
Travel to the place of full-time training is not included in the cost of training. Accommodation during full-time studies can be included in the full board tuition fees.
While Tecedu is not an accredited institution, we offer skills-based courses taught by real experts in their field, and every approved, paid course features a certificate of completion or attendance to document your accomplishment.
You can preview samples of the training materials and review key information about the course on our website. You can also review feedback and recommendations from students who already completed this course.
We want you to be happy, so almost all purchased courses can be returned within 30 days. If you are not satisfied with the course, you can request a refund, provided the request complies with our return policy.
The 30-day money back policy allows students to receive quality teaching services with minimal risk, we must also protect our teachers from fraud and provide them with a reasonable payment schedule. Payments are sent to instructors after 30 days, so we will not process refund requests received after the refund period.
We reserve the right, in our sole discretion, to limit or deny refund requests in cases where we believe there is refund abuse, including but not limited to the following:
- A significant portion of the course has been consumed or downloaded by a student before the refund was requested.
- Multiple refunds have been requested by a student for the same course.
- Excessive refunds have been requested by a student.
- Users whose account is blocked or access to courses is disabled due to violation of our Terms and Conditions or the Rules of Trust and Security.
- We do not grant refunds for any subscription services.
- These refund restrictions will be enforced to the extent permitted by applicable law.
We accept most international credit and debit cards like Visa, MasterCard, American Express, JCB and Discover. Bank Transfers also may be an option.
Conducting classes is based on the fact that the teacher demonstrates text, drawings, graphics, presentations on an interactive board, while the content appears in the student's electronic notebook. A specially designed digital notepad and pen are used to create and edit text and images that can be redirected to any surface via a projector.
Classes are live streamed online, automatically recorded and published on the Learning Portal, allowing you to save them for reuse anytime, anywhere, on any mobile device. This makes it possible not to miss classes and keep up with classes and keep up with the passage of new material.
Real-life training uses the principles of game organization, which allows future professionals to rehearse and hone their skills in a virtual emergency. Learning as a game provides an opportunity to establish a connection between the learning activity and real life.
The technology provides the following learning opportunities:
- Focused on the needs of the user
- Instant feedback
- Independent decision making and choice of actions
- Better assimilation and memorization of the material
- Adaptive pace of learning tailored to the individual needs of the student
- Better transfer of skills learned in a learning situation to real conditions
Basic principles of training:
- A gradual increase in the level of difficulty in the game;
- Using a simplified version of a problem situation;
- Action in a variable gaming environment;
- The right choice is made through experimentation.
The main advantages of Game Based Learning technology:
- Low degree of physical risk and liability
- Motivation to learn while receiving positive emotions from the process;
- Practice - mirroring the real situation
- Timely feedback
- Choice of different playing roles
- Learning in collaboration
- Developing your own behavior strategy
Conducting practical classes online using remote access technologies for presentations, multimedia solutions and virtual reality:
- Laboratory workshops that simulate the operation of expensive bench equipment in real production
- Virtual experiment, which is visually indistinguishable from a remote real experiment performed
- Virtual instruments, which are an exact copy of real instruments
- Mathematical modeling to clarify the physical characteristics, chemical content of the investigated object or phenomenon.
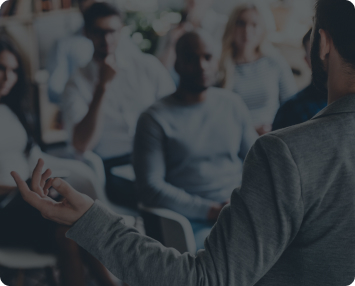